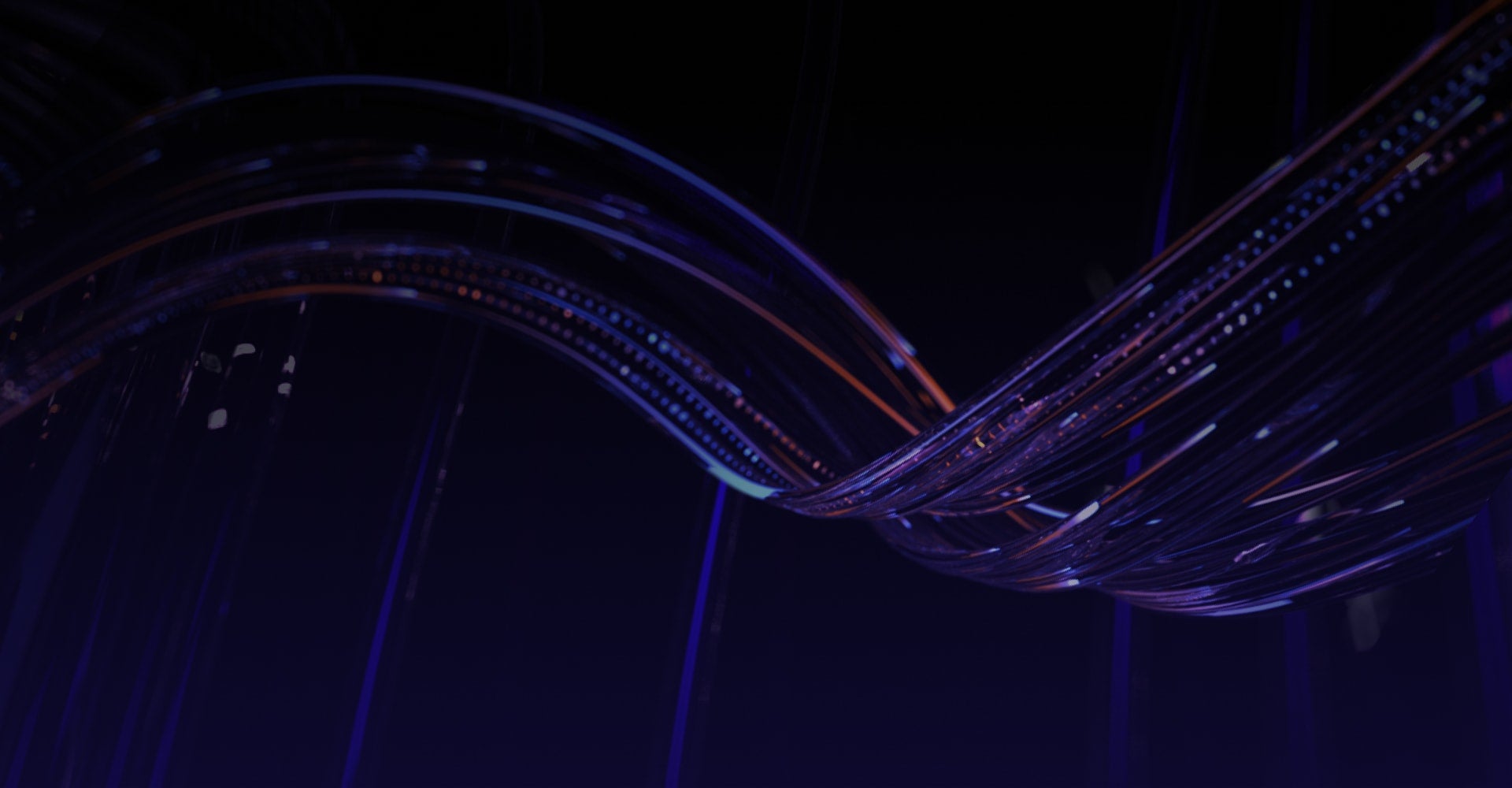
Product and Services Lifecycle Matrix
The Product and Services Lifecycle Matrix below highlights the lifecycle dates for the products or offerings that are being retired and will be subject to the phases outlined in Product Lifecycle Policies.
Please continue to reference this page to stay up to date on key announcements.
Product Lifecycle Dates
Offering | Notification of End of Life (NEOL) | End of Sale(EOS) | End of Support (EOSupp)* | End of Life(EOL) |
---|---|---|---|---|
DataRobot Pipelines | September 2022 | September 2022 | October 2022 | October 2022 |
Algorithmia | December 2022 | October 2022 | July 2023 | October 2023 |
Paxata | January 2023 | December 2022 | December 2023 | December 2023 |
Zepl | February 2023 | January 2023 | June 2023 | June 2023 |
Please refer the DataRobot Product Lifecycle Policy for more details on what each phase means
For any queries, contact us at support@datarobot.com or through the DataRobot Contact Us page.
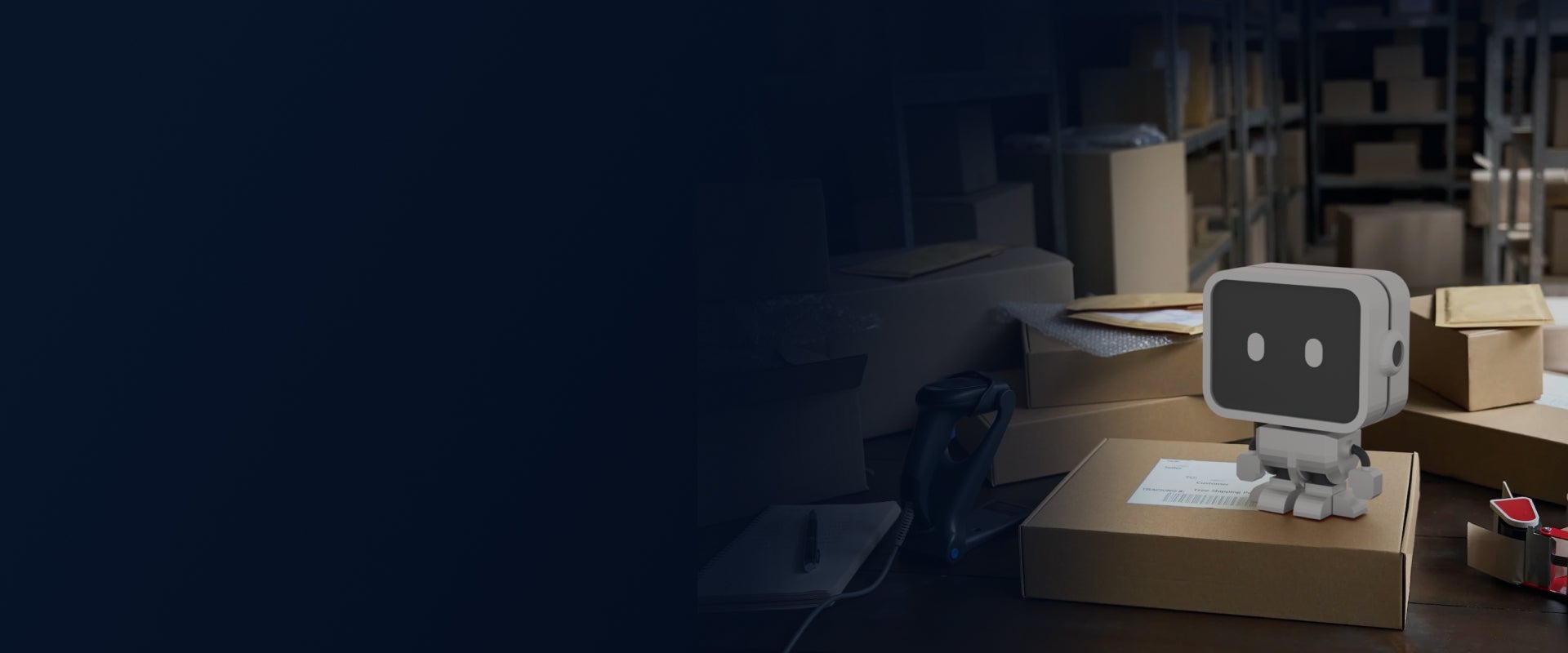